The potential of ML in transforming cancer diagnosis, prevention in healthcare is immense
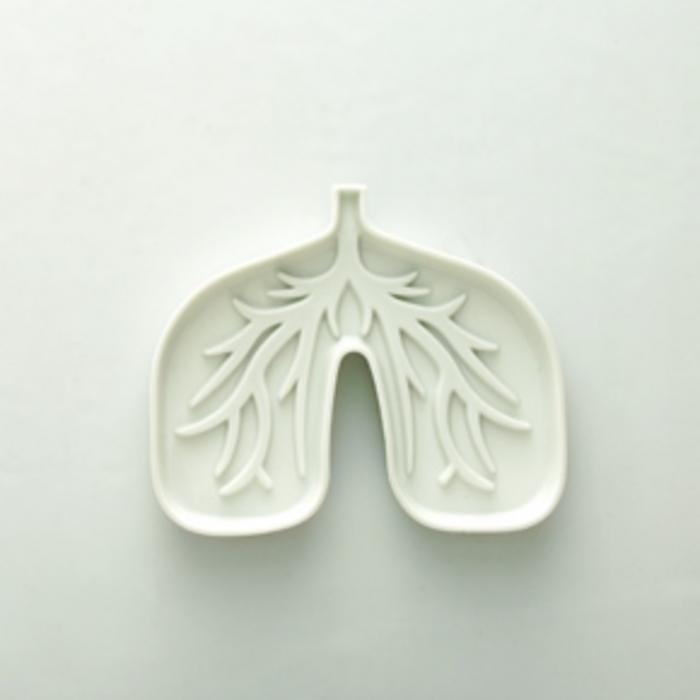
The utilization of machine learning in medicine has been a transformative development in many aspects. This innovative technology has enabled early detection of diseases and personalized treatment plans, pushing the boundaries of healthcare. In the field of cancer research, particularly in lung cancer screening, machine learning has once again taken center stage by simplifying and enhancing our understanding of who is at high risk.
Advancements in technology have always played a crucial role in improving patient care and outcomes in medicine. With the power of machine learning, there has been a significant breakthrough in assessing eligibility for lung cancer screening.
According to a recent study published on October 3rd in the open-access journal PLOS Medicine by Thomas Callender and colleagues from University College London, UK, a machine learning model equipped with data on age, smoking duration, and the number of cigarettes smoked per day can predict lung cancer risk and identify who needs lung cancer screening. Paper: https://journals.plos.org/plosmedicine/article?id=10.1371/journal.pmed.1004287
Cancer of the lungs is the leading cause of cancer-related deaths worldwide. It is difficult to survive without early detection. By screening those at a high risk of developing lung cancer, deaths due to the disease can be reduced by 25%. However, it is unclear how to determine the high-risk population. The current standard-of-care model for determining lung cancer risk requires 17 variables, most of which are not readily available in electronic health records.
In a recent study, researchers used data from the UK Biobank cohort (216,714 ever-smokers) and the US National Lung Screening Trial (26,616 ever-smokers) to develop new models of lung cancer risk. A machine learning model was used to predict a person's odds of developing and dying from lung cancer over the next five years, based on three predictors: people’s age, smoking duration and pack-years of smoking. The researchers tested the model on a third set of data from the US Prostate, Lung, Colorectal, and Ovarian Screening Trial. The model predicted lung cancer incidence and deaths with 83.9% and 85.5% sensitivity, respectively. All versions of the model had a higher sensitivity compared to currently used risk prediction formulas at an equivalent specificity.
Lung cancer remains the leading cause of cancer-related deaths worldwide. Detecting individuals at high risk is crucial for timely intervention and treatment. However, this process has traditionally been complicated and time-consuming, using multiple predictors and manual calculations.
Fortunately, parsimonious ensemble machine-learning models have simplified this approach. These models use just three key predictors to accurately determine an individual's eligibility for lung cancer screening.
The integration of machine learning in healthcare streamlines processes and improves accuracy by considering various factors such as age, smoking history, and family history of lung cancer. This enables healthcare professionals to prioritize resources efficiently and provide personalized care based on each patient's unique circumstances.
Machine learning algorithms learn from vast amounts of data available through electronic health records and other sources, continually adapting their predictions over time. This ensures they stay up-to-date with the latest research findings without requiring constant manual adjustments.
With machine learning paving the way for simplified risk assessment in lung cancer screenings, more lives can potentially be saved through early detection and intervention strategies. Identifying those at high risk sooner allows healthcare providers to offer targeted preventive measures, such as smoking cessation programs or further diagnostic tests when necessary.
Callender adds, “We know that screening for those who have a high chance of developing lung cancer can save lives. With machine learning, we’ve been able to substantially simplify how we work out who is at high risk, presenting an approach that could be an exciting step in the direction of widespread implementation of personalized screening to detect many diseases early.”
While further refinement and validation of these models are necessary, machine learning holds great promise for revolutionizing cancer diagnosis and prevention in healthcare practices.
As technology advances in the medical field, we must embrace these innovations responsibly while prioritizing patient well-being. Machine learning provides the opportunity to transform how we approach lung cancer.
In conclusion, the integration of machine learning into healthcare improves efficiency and has the potential to save lives. We must continue investing in research and innovation to improve our understanding of lung cancer risk factors and enhance our ability to detect them early on. Through collaborative efforts between healthcare professionals, researchers, policymakers, and technology experts, we can hope for a future where fewer lives are cut short by this devastating illness.