Deep learning now solves nanoparticle shape identification challenges
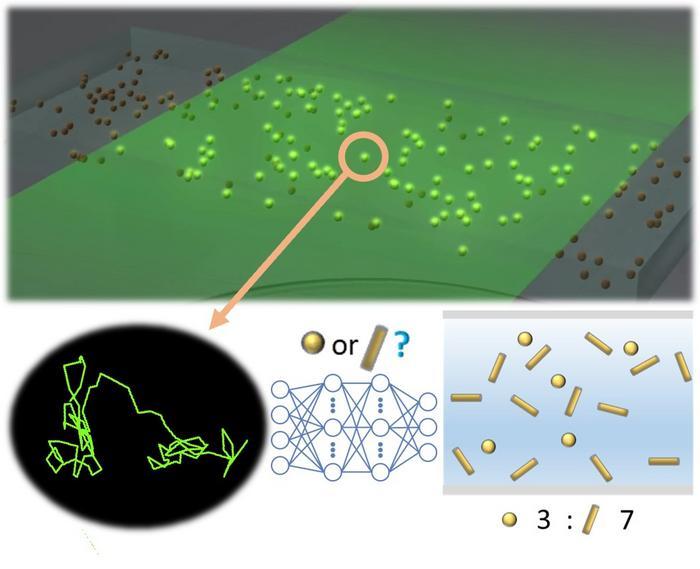
The Innovation Center of NanoMedicine (iCONM) and The University of Tokyo have proposed a new method to evaluate the shape anisotropy of nanoparticles. This new method solves a long-standing issue in nanoparticle evaluation that dates back to the time of Einstein. The method uses deep learning to detect differences in shape and has achieved an 80% classification accuracy on a single-particle basis for two types of gold nanoparticles that are approximately the same size but have different shapes. This innovative approach has the potential to advance fundamental research on Brownian motion of non-spherical particles in liquid. Furthermore, it can be useful in practical applications such as the detection of foreign substances in homogeneous systems.
The paper titled "Analysis of Brownian motion trajectories of non-spherical nanoparticles using deep learning" was published online in the APL Machine Learning journal on October 25, 2023. The group led by Prof. Takanori Ichiki, Research Director of iCONM (Professor, Department of Materials Engineering, Graduate School of Engineering, The University of Tokyo in Japan) proposed this new method.
Nanoparticles are useful materials in the medical, pharmaceutical, and industrial fields. Therefore, it is necessary to evaluate the properties and agglomeration state of each nanoparticle and perform quality control. Nanoparticle Tracking Analysis (NTA) is one way to evaluate nanoparticles in liquid by analyzing the trajectory of Brownian motion. It is a simple method to measure single particles from micro to nano size. However, it has a long-standing problem that it cannot evaluate the shape of nanoparticles.
Detecting the trajectory of Brownian motion is important as it reflects the influence of particle shape. However, measuring extremely fast motion is difficult, and conventional analysis methods are not accurate because they assume that the particle is spherical. Their research group has developed a deep learning model that identifies shapes from measured Brownian motion trajectory data without altering the experimental method.
Their model includes a 1-dimensional CNN model that extracts local features through convolution and a bidirectional LSTM model that accumulates temporal dynamics. By integrating these models, they have achieved approximately 80% classification accuracy on a single-particle basis for two types of gold nanoparticles that are approximately the same size but have different shapes. This is a significant improvement compared to conventional NTA alone.
Furthermore, they were able to create a calibration curve to determine the mixing ratio of a mixed solution of two types of nanoparticles (spherical and rod-shaped). This method is sufficiently accurate in detecting the shape of various types of nanoparticles in liquid using deep learning analysis, making it a practical tool for the first time.
In traditional NTA methods, it is not possible to directly observe the shape of particles, and the information obtained is limited. Although the trajectory of Brownian motion measured by the NTA device contains information on the shape of nanoparticles, detecting the shape anisotropy of nanoparticles has been a challenge due to the extremely short relaxation time. Also, conventional analysis methods assume particles to be spherical, which leads to inaccurate results when the particle is non-spherical. To overcome these challenges, they aimed to develop a new method that is simple and accessible. They introduced deep learning, which is good at finding hidden correlations in large-scale data, into data analysis without changing simple experimental methods. This approach enabled them to solve a long-standing problem in Brownian motion analysis and accurately detect the shape anisotropy of nanoparticles.
In this paper, they aimed to determine the shapes of two types of particles. However, they believe that the method used can have practical applications such as detecting foreign substances in homogeneous systems, considering the shapes of commercially available nanoparticles. The expansion of NTA can lead to applications not only in research but also in the industrial field. It can be useful in evaluating the properties, agglomeration state, and uniformity of non-spherical nanoparticles, and in quality control. This technology can be particularly helpful in evaluating the properties of diverse biological nanoparticles, such as extracellular vesicles, in an environment similar to that of living organisms. Furthermore, it has the potential to be an innovative approach in fundamental research on Brownian motion of non-spherical particles in liquid.