Experimental brain-like supercomputing system: Unlocking the potential of neuromorphic nanowire networks
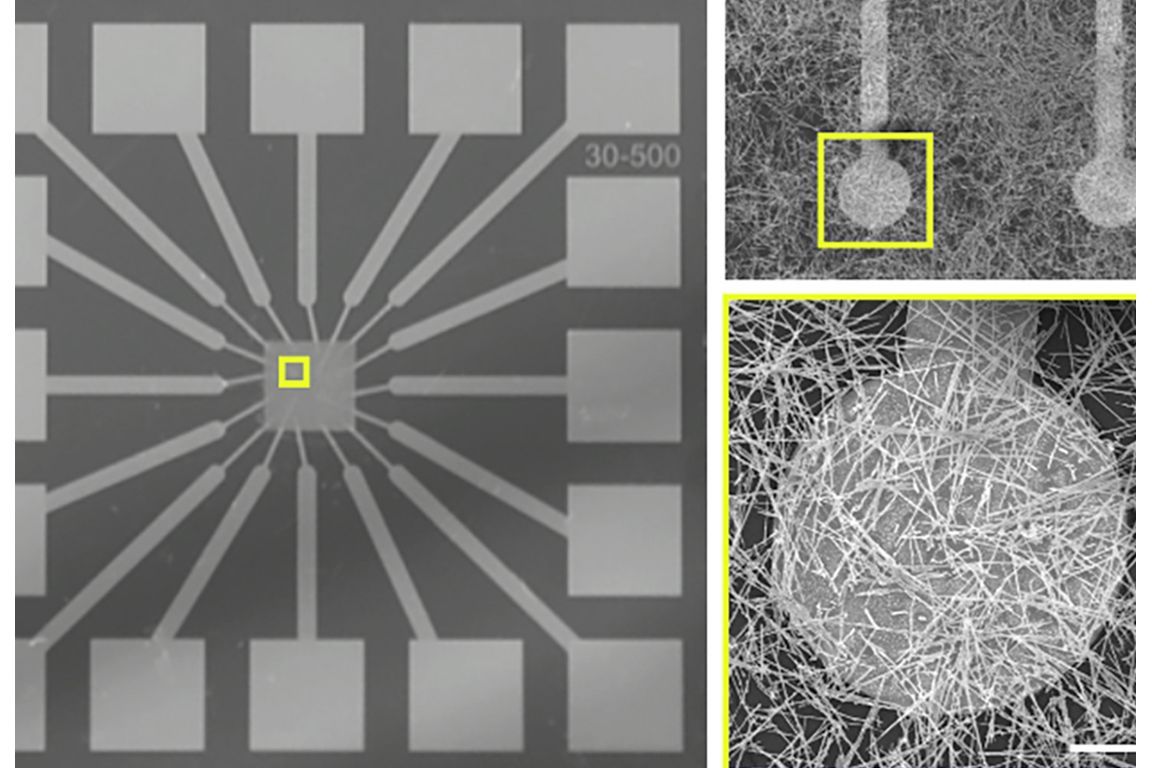
Scientists and researchers have been inspired by the intricacies of the human brain in creating advanced computing systems. The California NanoSystems Institute at UCLA has been at the forefront of developing a brain-inspired platform technology for computation. Their experimental computing system has shown remarkable potential in learning and identifying handwritten numbers with an impressive accuracy of 93.4%. This breakthrough is attributed to a novel training algorithm that provides continuous real-time feedback to the system, surpassing conventional machine-learning approaches.
The Brain-Like System: A Tangled Network of Nanowires
The brain-like computing system developed by researchers at UCLA and the University of Sydney is composed of a network of nanowires made of silver and selenium. These nanoscale wires self-organize into a complex, tangled structure on top of an array of electrodes. Unlike traditional computers, where memory and processing modules are separate entities, this nanowire network physically reconfigures itself in response to stimuli, with memory distributed throughout its atomic structure. The connections between wires can form or break, similar to the behavior of synapses in the biological brain.
Training the Brain-Like System with Handwritten Numbers
To train and test the brain-like system, researchers utilized a dataset of handwritten numbers provided by the National Institute of Standards and Technology. The images of these numbers were communicated to the system pixel-by-pixel using pulses of electricity, with varying voltages representing light or dark pixels. The streamlined algorithm developed by the University of Sydney allowed the system to process multiple streams of data simultaneously and adapt dynamically, leveraging its brain-like capabilities.
Real-Time Feedback: A Key to Enhanced Learning
The groundbreaking aspect of this experimental system lies in the real-time feedback provided during the training process. Unlike conventional approaches where training is performed after processing a batch of data, the brain-like system received continuous information about its success at the task as it learned. This constant feedback loop proved to be highly effective, resulting in an accuracy of 93.4% in identifying handwritten numbers. In comparison, a conventional machine-learning approach achieved an accuracy of 91.4%.
The brain-like computing system has some unique features that distinguish it from other computing approaches. One of these features is the system's distributed memory, which stores past inputs within the nanowire network. This embedded memory enhances the system's learning capabilities, making it highly accurate in identifying handwritten numbers.
Compared to silicon-based artificial intelligence systems, the brain-like system has the potential to operate with significantly lower power consumption. It can perform tasks that current AI systems find difficult, such as analyzing patterns in weather, traffic, and other dynamic systems.
The research team employed a co-design approach, developing both the hardware and software simultaneously. This approach ensures optimal integration between the brain-like system and its custom algorithm, resulting in enhanced performance. The combination of brain-like memory and processing capabilities embedded in physical systems with continuous adaptation and learning opens up new possibilities for edge computing.
Edge computing processes complex data on the spot without relying on communication with remote servers, making it suitable for various applications in robotics, autonomous navigation, smart devices, health monitoring, and more.
The brain-like computing system is still in the development phase, but its potential impact on various industries is immense. Its ability to perform complex tasks with lower energy consumption makes it an attractive alternative to traditional AI systems. The neuromorphic nanowire networks can unlock new opportunities in fields such as robotics, autonomous vehicles, the Internet of Things, and multi-location sensor coordination.
The experimental brain-like computing system represents a significant advancement in the field of neuromorphic computing. By harnessing the unique properties of nanowire networks and integrating them with custom algorithms, researchers have shown the potential for creating highly efficient and adaptable computing systems. As this technology continues to evolve, we can expect to see further breakthroughs in AI, edge computing, and various other domains, transforming the way we process and analyze complex data.
The study's corresponding authors include James Gimzewski, a distinguished professor of chemistry and member of the California NanoSystems Institute at UCLA, Adam Stieg, a research scientist and associate director of CNSI, Zdenka Kuncic, a professor of physics at the University of Sydney, and Ruomin Zhu, a doctoral student at the University of Sydney and first author of the study. Other co-authors include Sam Lilak, Alon Loeffler, and Joseph Lizier, all contributing to the research at UCLA and the University of Sydney.
The research was supported by the University of Sydney and the Australian-American Fulbright Commission. The brain-like computing system has some unique features that distinguish it from other computing approaches. One of these features is the system's distributed memory, which stores past inputs within the nanowire network. This embedded memory enhances the system's learning capabilities, making it highly accurate in identifying handwritten numbers.
Compared to silicon-based artificial intelligence systems, the brain-like system has the potential to operate with significantly lower power consumption. It can perform tasks that current AI systems find difficult, such as analyzing patterns in weather, traffic, and other dynamic systems.
The research team employed a co-design approach, developing both the hardware and software simultaneously. This approach ensures optimal integration between the brain-like system and its custom algorithm, resulting in enhanced performance. The combination of brain-like memory and processing capabilities embedded in physical systems with continuous adaptation and learning opens up new possibilities for edge computing.
Edge computing processes complex data on the spot without relying on communication with remote servers, making it suitable for various applications in robotics, autonomous navigation, smart devices, health monitoring, and more.
The brain-like computing system is still in the development phase, but its potential impact on various industries is immense. Its ability to perform complex tasks with lower energy consumption makes it an attractive alternative to traditional AI systems. The neuromorphic nanowire networks can unlock new opportunities in fields such as robotics, autonomous vehicles, the Internet of Things, and multi-location sensor coordination.
The experimental brain-like computing system represents a significant advancement in the field of neuromorphic computing. By harnessing the unique properties of nanowire networks and integrating them with custom algorithms, researchers have shown the potential for creating highly efficient and adaptable computing systems. As this technology continues to evolve, we can expect to see further breakthroughs in AI, edge computing, and various other domains, transforming the way we process and analyze complex data.
The study's corresponding authors include James Gimzewski, a distinguished professor of chemistry and member of the California NanoSystems Institute at UCLA, Adam Stieg, a research scientist and associate director of CNSI, Zdenka Kuncic, a professor of physics at the University of Sydney, and Ruomin Zhu, a doctoral student at the University of Sydney and first author of the study. Other co-authors include Sam Lilak, Alon Loeffler, and Joseph Lizier, all contributing to the research at UCLA and the University of Sydney.
The research was supported by the University of Sydney and the Australian-American Fulbright Commission.