Japanese researchers accelerate the phase identification of multiphase mixtures with deep learning
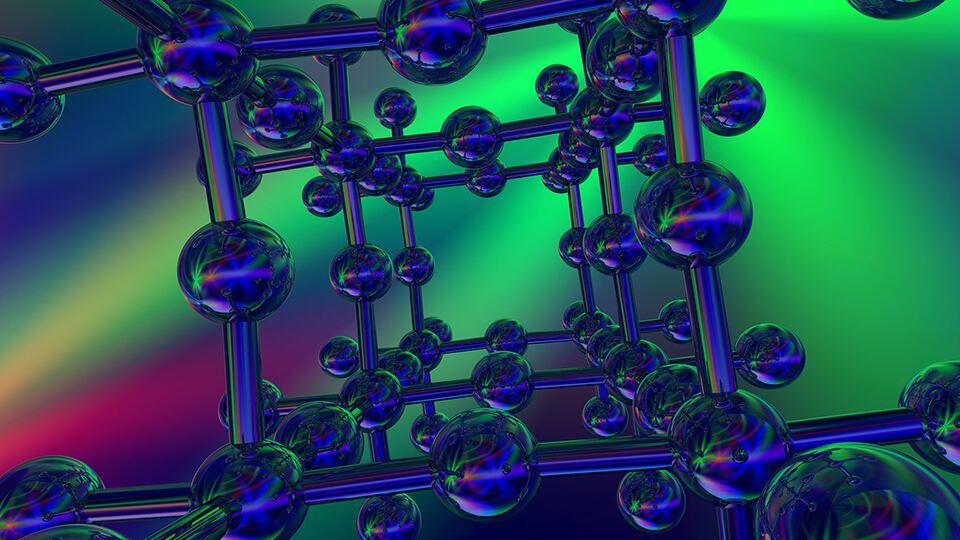
Crystalline materials are crucial components in various industries such as semiconductors, pharmaceuticals, photovoltaics, and catalysts. These materials have an ordered, three-dimensional structure made up of atoms, ions, or molecules. As scientists continue to design novel materials to address emerging challenges, the need for precise identification methods becomes increasingly essential. Powder X-ray diffraction is the most widely used method to identify the structure of crystalline materials. However, accurately identifying different types of crystals in multiphase samples can be complex and time-consuming.
To expedite the phase identification process, researchers have turned to innovative data-driven methods, such as machine learning. While substantial progress has been made in utilizing machine learning for known phases, identifying unknown phases in multiphase samples remains a challenge. In a recent study published in the Advanced Science journal, researchers from Tokyo University of Science, National Defense Academy, National Institute for Materials Science, Tohoku University, and The Institute of Statistical Mathematics proposed a deep learning model that can detect a previously unknown quasicrystalline phase present in multiphase crystalline samples.
The Role of Deep Learning in Phase Identification
Deep learning is a subset of machine learning that involves training artificial neural networks with multiple layers to learn patterns and make predictions. In the context of phase identification, deep learning can be used to analyze X-ray diffraction patterns and distinguish different phases within multiphase samples. This approach offers the potential to significantly reduce the time and effort required for accurate identification.
Developing the Deep Learning Model
To develop their deep learning model, the researchers created a "binary classifier" using 80 types of convolutional neural networks. They trained the model using synthetic multiphase X-ray diffraction patterns that represented the expected patterns associated with the icosahedral quasicrystal (i-QC) phase. The model's performance was assessed using both synthetic patterns and a database of actual patterns. Remarkably, the model achieved a prediction accuracy of over 92%.
Successful Identification of Unknown Phases
The researchers tested their deep learning model on multiphase Al-Si-Ru alloys, which contained an unknown i-QC phase. The model successfully identified the presence of the unknown i-QC phase when screening 440 measured diffraction patterns from unknown materials in six different alloy systems. The presence of the i-QC phase was further confirmed through microstructure and composition analysis using transmission electron microscopy. Importantly, the model was able to identify the i-QC phase even when it was not the most prominent component in the mixture.
Identifying new structures with the proposed deep learning model goes beyond identifying i-QC phases. It also has the potential to identify decagonal and dodecagonal quasicrystals (QCs) and can be applied to various crystalline materials. This makes the model versatile in accelerating the phase identification process of multiphase samples in a wide range of industries.
This deep learning model is a significant breakthrough for materials science. The model enables researchers to identify unknown quasicrystalline phases efficiently and explore the potential applications of these materials. The discovery of new materials with enhanced properties for energy storage, carbon capture, and advanced electronics is possible with this advancement.
The success of the deep learning model in identifying unknown phases showcases the potential of artificial intelligence and machine learning in accelerating scientific research. As researchers refine and expand upon these methods, the identification of complex multiphase mixtures will become faster and more accurate. This approach will streamline materials development processes and pave the way for discoveries and advancements in various scientific fields.
In summary, the development of a deep learning model for the rapid identification of unknown quasicrystalline phases in multiphase samples is a significant milestone in materials science. By harnessing the power of artificial intelligence and machine learning, researchers have an innovative approach to overcome the challenges associated with complex phase identification. This approach has the potential to revolutionize industries reliant on crystalline materials, leading to the discovery of new and improved materials for a wide range of applications. As the field of deep learning continues to advance, we can expect further breakthroughs in phase identification and materials research.