Study casts doubts on the reliability of machine learning methods
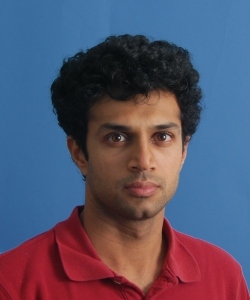
In today's digital era, machine learning plays a vital role in our lives by driving social media expansion and shaping various scientific research fields. However, a recent study by UC Santa Cruz has raised concerns about the reliability of widespread machine learning methods behind link prediction.
Link prediction is a popular machine learning task that evaluates the links in a network and predicts future connections. From suggesting friends on social media to predicting the interaction between genes and proteins, link prediction has become a benchmark for testing the performance of machine learning algorithms. But is it trustworthy?
The study by UC Santa Cruz Professor of Computer Science and Engineering C. "Sesh" Seshadhri, in collaboration with Nicolas Menand, reveals the flaws in evaluating the accuracy of link prediction. The commonly used metric for measuring link prediction performance, known as AUC, fails to capture crucial information, thereby giving an exaggerated sense of success.
Seshadhri, a respected figure in theoretical computer science and data mining, discovered mathematical limitations hindering the performance of machine learning algorithms. His investigation into link prediction revealed that the seemingly impressive results may not reflect reality. According to Seshadhri, "It feels like if you measured things differently, maybe you wouldn't see such great results."
Low-dimensional vector embeddings are the key to link prediction, a process that represents individuals within a network as mathematical vectors in space. However, the study finds that AUC, the most commonly used metric, fails to account for fundamental mathematical limitations. This ultimately creates an inaccurate measure of link prediction performance.
The study's findings cast doubt on the widespread use of low-dimensional vector embeddings in machine learning, challenging the notion that these methods are as effective as previously thought. Seshadhri and Menand introduced a new metric, VCMPR, to capture the limitations more comprehensively. Interestingly, when using VCMPR, most leading methods in the field performed poorly. This calls into question the reliability of these algorithms.
Beyond the immediate concern for machine learning accuracy, this research has broader implications for trustworthiness and decision-making in machine learning. Using flawed metrics to assess performance could lead to flawed decision-making in real-world machine-learning applications. Seshadhri asks, "If you have the wrong way of measuring, how can you trust the results?"
While some may argue that these findings are not surprising to those deeply entrenched in the field, the wider community of machine learning researchers needs to take note of this skepticism. The study challenges the dominant philosophy within machine learning, urging researchers to question the validity of metrics and strive for a more comprehensive understanding of their experiments.
In a world where machine learning extends beyond its domain and significantly impacts various fields such as biology, accuracy and trustworthiness are paramount. Biologists utilizing link prediction to identify potential protein interactions in drug discovery, for instance, heavily rely on the expertise of machine learning practitioners to produce reliable tools.
This study, funded by the National Science Foundation and the Army Research Office, serves as a cautionary tale for the machine learning community. It reminds us of the need to approach research with skepticism and constantly question the accuracy of our methodologies. True progress lies in the pursuit of a deeper understanding rather than just chasing higher scores on flawed metrics.
As the field of machine learning continues to evolve, researchers and practitioners must consider diverse perspectives, challenge conventional wisdom, and prioritize the development of more accurate and trustworthy methods. Only then can we fully harness the potential of machine learning while ensuring its reliability and impact on society?