Simulations aid breakthroughs in predicting catalyst performance for fuel cells
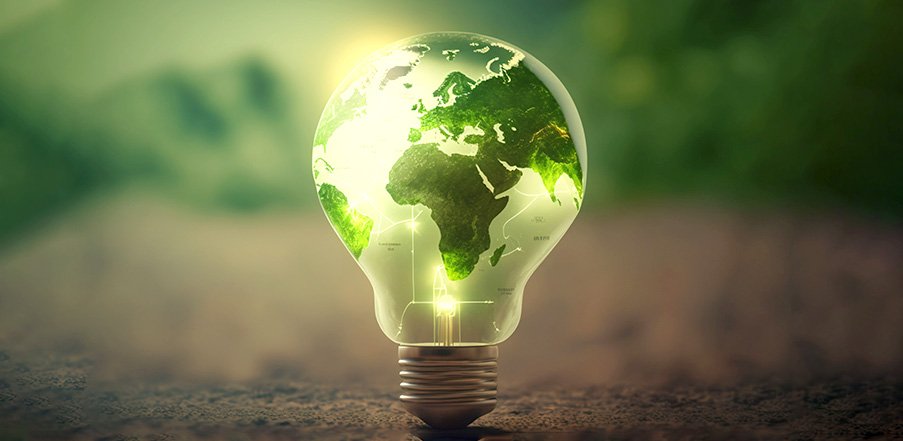
Japanese scientists from Tohoku University have made significant advances in predicting the performance of catalysts for fuel cells using state-of-the-art supercomputer simulations. This breakthrough holds great promise for accelerating the development of efficient catalysts and advancing clean energy technologies.
Fuel cell technology has long been hailed as a promising solution for clean energy. However, the efficiency of catalysts has remained a major challenge impeding their widespread adoption. To address this issue, researchers have focused on molecular metal-nitrogen-carbon (M-N-C) catalysts, which exhibit unique structural properties and exceptional electrocatalytic performance, particularly in the oxygen reduction reaction (ORR) of fuel cells. These catalysts offer a cost-effective alternative to platinum-based catalysts commonly used.
Among the M-N-C catalysts, a particular variant called metal-doped azaphthalocyanine (AzPc) holds great potential. These catalysts possess distinctive structural properties characterized by elongated functional groups. When placed on a carbon substrate, they assume intricate three-dimensional configurations, resembling a dancer setting foot on a stage. The structural changes caused by these catalysts influence their effectiveness in the ORR, particularly at different pH levels.
However, translating these advantageous structural properties into improved catalyst performance requires extensive modeling, validation, and experimentation, which can be resource-intensive. To overcome this challenge, researchers at Tohoku University turned to supercomputer simulations to study how the performance of carbon-supported Fe-AzPcs catalysts for oxygen reduction reactions varies with different pH levels and the interaction between electric fields and surrounding functional groups.
Lead author Hao Li, an associate professor at Tohoku University's Advanced Institute for Materials Research, stated, "By incorporating large molecular structures with complex long-chain arrangements, or 'dancing patterns,' containing over 650 atoms, we were able to analyze the performance of Fe-AzPcs in the ORR."
The crucial aspect of the research was the close matching between the pH-field coupled microkinetic modeling and the observed efficiency of the ORR, as confirmed by experimental data. Li added, "Our findings indicate that evaluating the charge transfer occurring at the Fe-site, where the Fe atom typically loses approximately 1.3 electrons, could serve as a useful method for identifying suitable surrounding functional groups for ORR. Essentially, we have created a direct benchmark analysis for the microkinetic model to identify effective M-N-C catalysts for ORR under different pH conditions."
This breakthrough in utilizing supercomputer simulations to predict catalyst performance brings a significant boost to fuel cell development. By reducing the time and resources required for iterative experimental testing, researchers can now focus on designing and developing efficient catalysts for both alkaline and acidic environments, further advancing clean energy solutions.
The research team's publication titled "Benchmarking pH-Field Coupled Microkinetic Modeling Against Oxygen Reduction in Large-Scale Fe-Azaphthalocyanine Catalysts" highlights the collaborative efforts of several scientists, including Di Zhang, Yutaro Hirai, Koki Nakamura, Koju Ito, Yasutaka Matsuo, Kosuke Ishibashi, Yusuke Hashimoto, Hiroshi Yabu, and Hao Li.
As these simulations continue to evolve and more accurate predictions are made, the field of catalyst development for fuel cells is expected to progress exponentially. The ability to harness clean and efficient energy from fuel cells brings us closer to a sustainable future and reduces our dependence on fossil fuels.